Advances in earthquakes forecasting pass through machine learning
In today’s interview, we meet Dr Fabio Corbi, a researcher at IGAG-CNR, the Institute of Environmental Geology and Geoengineering of the Italian National Research Council. Fabio has experience in analogue modelling of megathrust earthquakes and he is currently exploring the potentiality of machine learning in this research field.
Hi Fabio, would you briefly tell us about you and your career?
Ciao Gabriele, first of all, thank you so much for this interview. I’m a geologist with a PhD in geodynamics. My career as a young researcher started at Roma Tre University, Italy, in 2009. During my post-docs, I moved to GFZ-Potsdam, Germany, then to Montpellier University, France, and finally back again to Roma Tre University. This mobility gave me the chance to interact and learn from different brilliant researchers, who helped me to develop a strong multidisciplinary approach that I consider very useful in my research.
My research mainly aims at understanding subduction megathrust earthquakes and their relationship with other geophysical parameters. During my PhD, I had the chance to successfully start a new seismo-tectonic analogue modelling research line, which was baptized ‘gelquakes’ (Figure 1; see also https://aspsync.wordpress.com/gelquakes/). This model is capable of reproducing the basic characteristics of the seismic cycle of subduction megathrusts. The gelquakes apparatus has been used for investigating a variety of megathrust earthquakes related topics such as asperities synchronization and the correspondence between interseismic coupling and slip pattern. I then became a Machine Learning enthusiast a few years ago, after applying basic algorithms for the prediction of slip phases occurrence in my experiments.
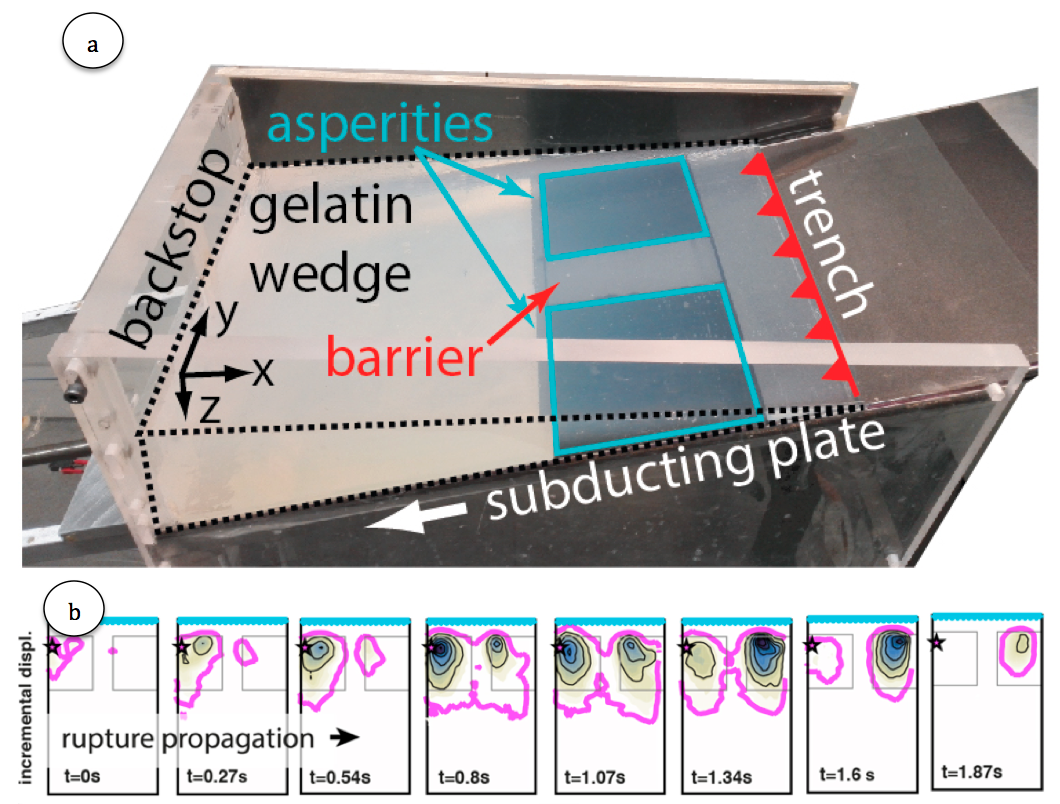
Figure 1: Panel (a) shows an oblique view of one of the models used for studying asperities synchronization. The megathrust embeds two asperities of equal size and friction (modified from [3]). Panel (b) shows incremental maps of surface displacement associated with a synchronized asperities rupture. Time since the beginning of the gelquake is reported in each panel.
How do you think Machine Learning can help understanding faulting and earthquakes?
Machine Learning (ML) is providing a great input for seismic hazard assessment and for understanding faulting, starting from laboratory experiments. Rouet-Leduc and coworkers in 2018 showed that in a relatively simple laboratory fault analogue, ML can predict the earthquake timing. The prediction is accomplished by analysing and interpreting a series of characteristics, or features, hidden in acoustic emissions generated by a fault gouge material undergone a direct shear, which represents tectonic loading in nature.
The work of Rouet-Leduc started a new wave of studies aimed at understanding and predicting fault seismic behaviour using ML. We can say that three main research branches of applied ML for seismic hazard are active at the moment: 1) laboratory geodesy, 2) laboratory seismology, and 3) field studies. The latter is showing the great potential of ML to detect and characterize earthquakes, precursors to earthquakes (foreshocks and slow slip), and aftershocks..
So ML has great potential in the broad field of seismic hazard, but what contribution does ML give to your research in particular?
I think I’ve been lucky to be in the right place and at the right time when my friends FG and JB asked: “why don’t you use ML in your experiments, Fabio?”. This is the way I started playing with ML algorithms. In the beginning, I asked ML to predict the time to fault failure in a relatively simple experiment. I then used the intuition that the displacement field I was measuring with my video camera was equivalent to a dense, homogeneously spaced geodetic network, which is why gelquakes are nowadays also known as laboratory-geodesy experiments [2]. And with the help of a group of colleagues, we demonstrated that ML predicts the timing and size of analogue earthquakes by deciphering the spatially and temporally complex surface deformation history (Figure 2). I’m very happy to note that this paper was within the top downloaded papers in 2018-2019 in the journal Geophysical Research Letters.

Figure 2: Space-time reconstruction of observed analogue earthquakes defined by the red squares, and the time to failure predicted by ML, defined by the coloured background shading. The blue-ish colour bar indicates an analogue earthquake is imminent at a given ‘latitude’ of the experimental subduction zone. This figure demonstrates that ML, by deciphering the deformation history measured by ‘virtual GPS stations’, can predict the timing and the size of slip phases in laboratory experiments.
Congratulations! That’s a success and it shows the wide interest in the topic and the relevance of your work within it.
Thank you! But, to go back to the research, it is easy to note that the ideal monitoring methodology implemented in my experiment doesn’t exist in nature. So, I thought it was necessary to investigate the minimum prerequisite (in terms of data availability) needed for ML to start making proper predictions. In fact, in a second ML-based work, I investigated how the space-time coverage of geodetic data influences the performances of a binary classifier trained to predict analogue earthquakes imminence. We found which region produces the most important geodetic information for the imminence classification and that the definition of ‘alarm’ (in other words, the amount of time that we consider for an event to be imminent) is probably more important than the geodetic record length or the number of stations available. These results represent useful hints for potential progress in earthquake prediction also in nature. Slow slip episodes, given their shorter recurrence time and similar scaling [5] with respect to regular mega-earthquakes, occurring, for example along the Cascadia subduction zone, are potentially good candidates for testing whether geodesy-feed ML can predict the timing and the size of upcoming events.
It sounds all very exciting, Fabio. What are your plans for the future?
I learned to be flexible in terms of planning scientific research. For sure, I’d like to continue studying megathrust earthquakes and invest more time in the development of novel seismotectonic experiments, improving the monitoring techniques and maximizing the lesson we can learn from them. I have the feeling that for some aspects, for example, the 3D nature of our setup, experiments could be a bit ahead with respect to the equivalent numerical models and therefore it could be smart to profit from this advantage. I have many ideas – not only related to ML – that I’d like to test in the near future and I’m convinced that by involving more researchers from different disciplines we can still learn a lot from laboratory experiments.